21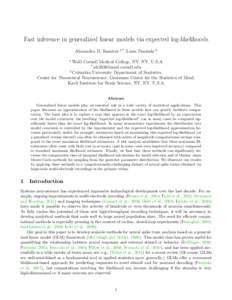 | Add to Reading ListSource URL: www.stat.columbia.eduLanguage: English - Date: 2013-06-06 11:45:10
|
---|
22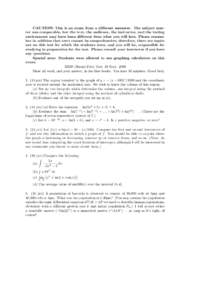 | Add to Reading ListSource URL: www.math.niu.eduLanguage: English - Date: 2000-09-29 19:49:49
|
---|
23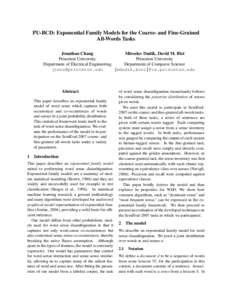 | Add to Reading ListSource URL: wordnet.cs.princeton.eduLanguage: English - Date: 2007-07-08 21:39:23
|
---|
24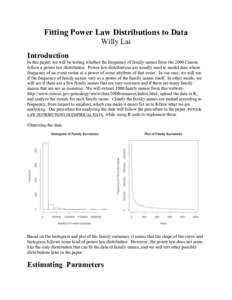 | Add to Reading ListSource URL: www.stat.berkeley.eduLanguage: English - Date: 2012-05-03 16:56:38
|
---|
25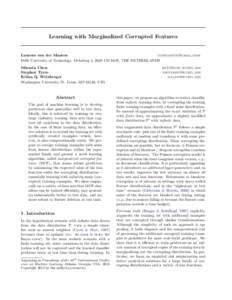 | Add to Reading ListSource URL: lvdmaaten.github.ioLanguage: English - Date: 2016-07-16 15:30:43
|
---|
26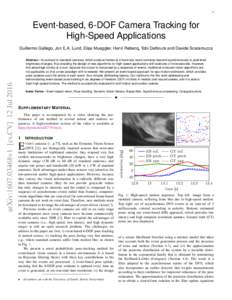 | Add to Reading ListSource URL: rpg.ifi.uzh.chLanguage: English - Date: 2016-07-15 03:40:35
|
---|
27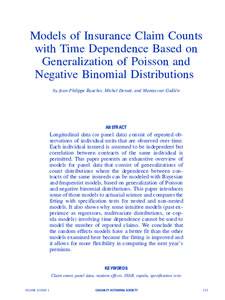 | Add to Reading ListSource URL: www.variancejournal.orgLanguage: English - Date: 2008-06-30 10:20:05
|
---|
28 | Add to Reading ListSource URL: jmlr.orgLanguage: English - Date: 2016-06-06 23:29:35
|
---|
29 | Add to Reading ListSource URL: jmlr.orgLanguage: English - Date: 2016-05-01 21:17:10
|
---|
30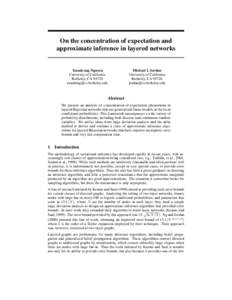 | Add to Reading ListSource URL: dept.stat.lsa.umich.eduLanguage: English - Date: 2004-01-18 19:14:54
|
---|